This Is How to Get Started With AI When the Only Thing You Know Is the Acronym AI integration can't happen overnight, and it won't happen at all unless you initiate the process.
By Sourav Dey Edited by Dan Bova
Opinions expressed by Entrepreneur contributors are their own.
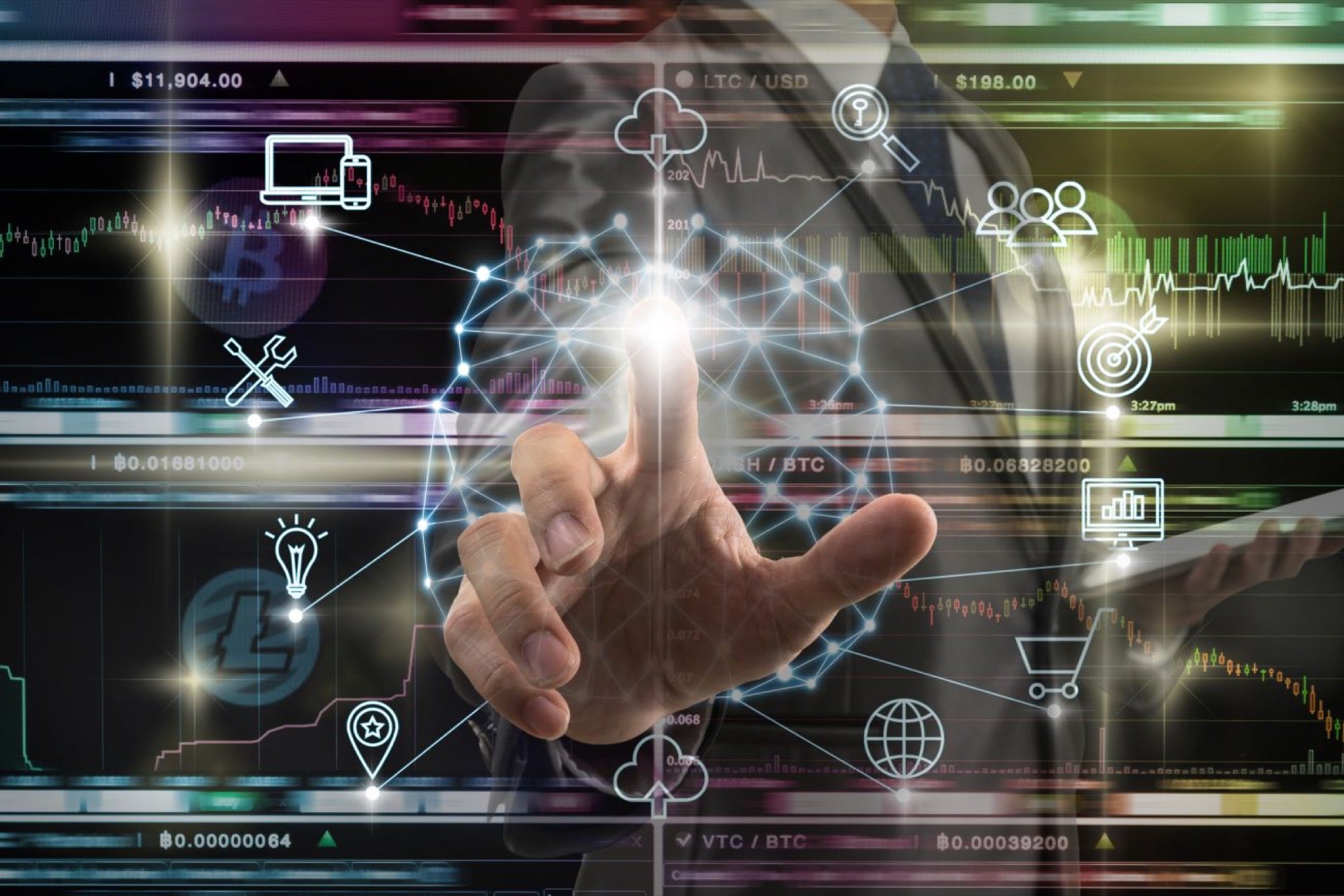
Unless you've been living under a rock, you've heard the buzz around artificial intelligence. So it might surprise you to learn that, according to a 2017 survey published by McKinsey Global Institute, out of 3,000 AI-aware executives, only one in five are using any AI-related technology in core areas of their businesses.
Why aren't entrepreneurs and executives jumping on what they know to be a market-changing technology? In a word, uncertainty. With AI still young, leaders aren't sure where to apply it, how to ensure a return on their investment or, most of all, how to implement it.
To be sure, AI can be intimidating. It's not a switch you flip or a widget you jam into your product. Winning with AI requires serious analysis about where to play and how to win, backed by rapid investments in software, data and machine learning.
Related: Ready or Not, It's Time to Embrace AI
So where to start? We like to think about AI at three levels of granularity: business patterns, core technologies and use cases. Because virtually all business applications of AI fall within three patterns, examining them is a great way to understand AI's use cases and specific technologies.
Recurring business patterns
AI describes a family of technologies that, in a rough sense, can be compared to a human brain. While AI might never replicate the creative problem-solving that people are capable of, it does offer enormous processing power and decision-making skills. Practically speaking, it'll never get bored, get tired or call in sick the Monday after the Super Bowl. The advantages of AI mean that, in certain situations, it can perform work in mere minutes that would take humans thousands of hours.
Related: The 5 Most Interesting Artificial Intelligence Trends for Entrepreneurs to Follow in 2018
AI as triage, the pattern representing roughly half our work at Manifold, serves to augment the decision-making ability of human beings. Instead of having a security guard watch hours of surveillance footage, an AI solution can flag instances of suspicious activity, greatly reducing the human workload. AI as triage is especially advantageous because the software doesn't need to be 100 percent accurate. Even an algorithm that's 80 percent accurate saves an incredible amount of time; plus, in many cases, its accuracy improves with use.
Entity resolution and topic modeling are sophisticated examples of AI as an organizer, but this AI solution essentially involves labeling data assets in order to unlock the insights they can provide. As big data takes over, AI will be essential to managing and manipulating mass quantities of information.
As triage and organizer, AI requires a degree of human input and direction. Autonomous AI, however, is capable of learning certain tasks that require complex decisions to be made. Autonomous AI often receives the most attention because it makes innovations like self-driving cars possible, but at least in the short term, the business world might have more use for AI's organizational and triage capabilities.
Whatever you choose to do with AI, don't delay. "AI is going to revolutionize the world more than any other tech advancement of the past 30 years," Mark Cuban remarked at the recent Upfront Summit in Los Angeles. Like the internet, AI will produce a rich-get-richer environment, and those who get a head start are going to run the table. So far, the following areas of AI show particular business promise:
1. Predictive analytics
Imagine being able to project a customer's worth as soon as he buys your service. Sounds impossible, right? Well, in the course of our AI-as-triage work, we helped a leading online registry predict the lifetime value of its patrons within a few days of sign-up with 90 percent accuracy. Now, the registry can make more informed decisions about its customer service, delivering as much value as possible to its most loyal users.
Of course, creating a predictive solution requires a complete record of your customer interactions. Building this database takes time, but many of the necessary components are available off the shelf. Even if predictive analytics are a way off for your business, start collecting customer data now so you'll have it when you decide to tap into AI.
2. Computer vision
What started as a cost-prohibitive technology has become commonplace in everyday life. Computer vision is the leap between storing images and videos to understanding their contents. Every time your photo app recognizes your face or you deposit a check via a mobile device, you're using computer vision. If your business works with unstructured visual data in almost any way, it can take advantage of this type of AI.
Related: Can Artificial Intelligence Identify Pictures Better Than Humans?
If you're stuck, think about all the corners of the market where computer vision is cropping up. Self-driving cars use it to detect road lines, pedestrians and other cars. Twitter uses it to crop photos uploaded by users to their most interesting parts. Computer vision can automatically label photos and videos, and Google's Image Search uses this technology to index all images on the internet.
3. Natural language processing
Computers haven't just become better at understanding visual data; they've started to master the intricacies of human speech and writing via natural language processing. In short, NLP algorithms decode information contained within free text. With deep learning, NLP can search huge quantities of text for both specific information and areas of contextual relevance.
Acting as triage, AI can look through medical records and determine what to code for. Apixio, for example, is using NLP to locate and "read" charts across electronic health records systems, standalone images and more. According to a study it conducted, Apixio's AI-fueled platform was 20 percent more accurate and 400 percent more productive than typical approaches. Similarly, NLP can comb through legal documents to find ones that are relevant to a specific case.
Much like computer vision, using NLP requires identifying information that you would like to retrieve or alter in large chunks of text. Examples include customer feedback, media mentions of your company, search queries and internal records. For instance, to resolve a health IT client's entity resolution problem, we built an AI solution using NLP that ingests a large collection of records, identifies duplicates and merges them into master records. Without automation, the client would have spent thousands of man hours reviewing and organizing those millions of records.
Whatever you do, AI integration won't happen overnight -- but it won't happen at all unless you initiate the process proactively. Start by identifying opportunities for quick business wins to build momentum and ensure future AI investments are on solid ground. Curate and prioritize your opportunities based on the complexity of implementation and the significance of the return. AI is often lauded for its ability to reduce costs, but its potential to drive growth matters just as much.
Your next task? Start thinking about how you'll collect, store, transform and label key data assets to power your AI solution. You'll need them organized and updated to properly screen the opportunities you spot. Fortunately, once you've got your data in shape, you'll have the hardest part behind you.