Here's How AI Plays a Pivotal Role in Assessing Loan Seekers With no Credit History AI has revolutionized almost every business sector and has made deep inroads into the BFSI sector
By Rohit Garg
Opinions expressed by Entrepreneur contributors are their own.
You're reading Entrepreneur India, an international franchise of Entrepreneur Media.
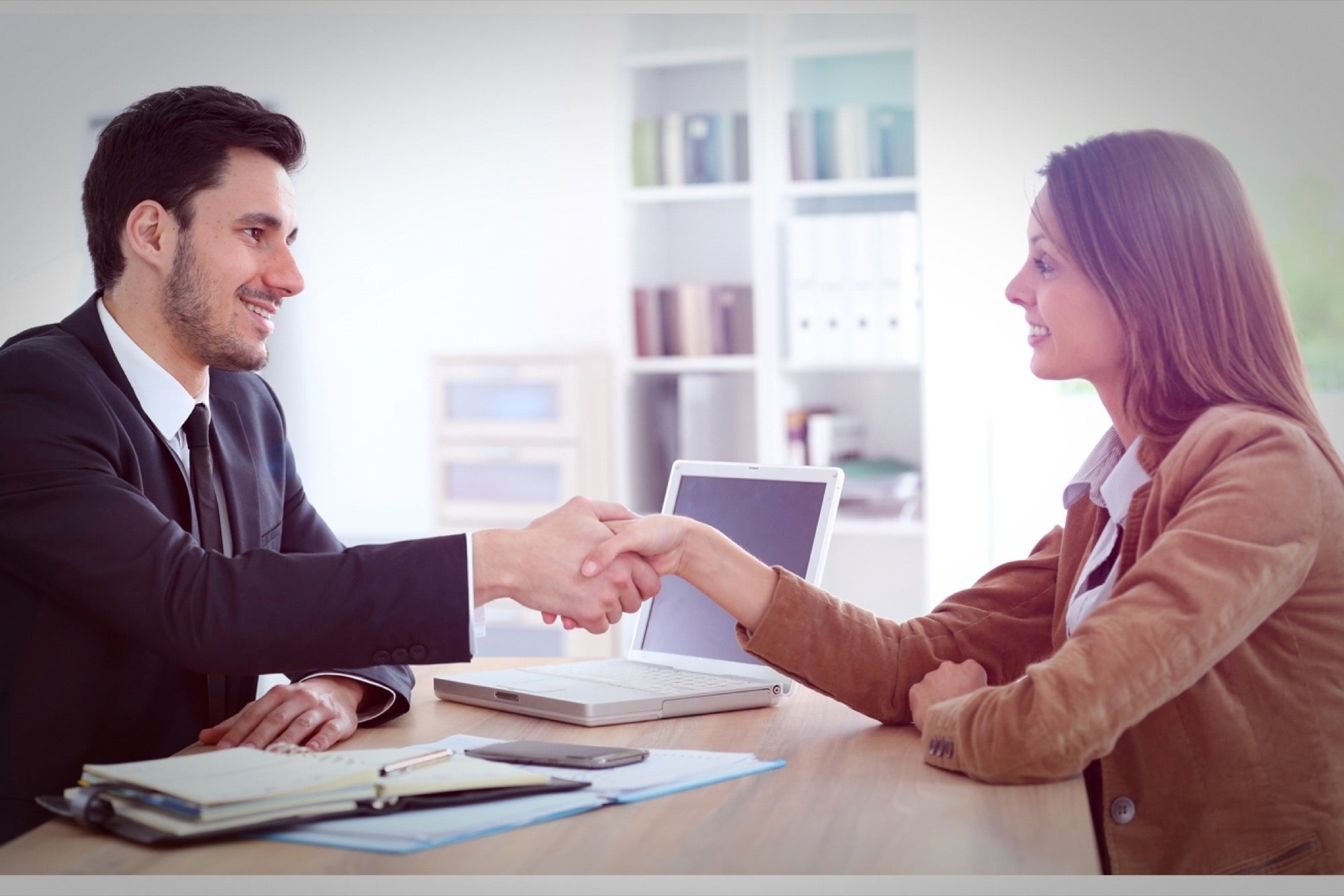
AI has been a game changer in the lending space which has existed in its current form for the past few decades. In its core, credit disbursal requires an accurate understanding of the customer profile and then allocating the appropriate loan terms. However, the process has been rife with manual due diligence, tedious paperwork taking days and dependence on legacy systems that are largely reliant on credit bureau data (Which is restricted to <20per cent of the population in India).
Solving Issues
Artificial Intelligence has solved multiple problems faced by legacy systems in making real-time credit decisions using new age alternate data through different use cases. We can broadly categorize the implementation of AI in a loan cycle for fraud borrower identification, estimation of the user's earning capacity and prediction of repayment intent. While traditional financial firms and even most fintech firms rely on field forces to do user authentication, AI models can be used to carry out fraud detection remotely with a high degree of accuracy. Increases reach of fintech lenders to areas far and wide through mobile app-based solutions.
Based on permissions granted by the user to these credit-facilitating apps, thousands of data points like text and call logs, GPS data, address books and digital transactions, etc can be extracted in a secure and encrypted manner. AI models can then create user's earning and risk profile by aggregating behavioural traits and smartphone habits. While previous attempts at loan process automation were GIGO systems ( "garbage-in-garbage-out") depended heavily on the quality of input data, modern AI algorithms have circum-navigated these issues.
The Success Story
One oft-quoted success story for AI model is in rating customers that maintain their earnings to expense ratio but are stricken by uneven cash flows. E.g. people involved in blue/grey collar jobs or microentrepreneurs like cab drivers have volatile monthly earnings with barely enough buffer for month-end liquidity. Similarly, small shops face a cash crunch due to inventory mismatch. AI models can use historical sales cycle and inventory requirement to predict the payment capacity and cash flow timings of the borrower making a scope for customized loan amount and tenure.
Though established financial firms have started adopting AI tools, USP of modern lending startups lies in their rapid prototyping and execution of the latest AI algorithms to keep up the latency with rising data. e.g. Logistic regression has been the pinnacle of classification algorithms deployed for financial risk assessment. However, there are tonnes of manual data pre-processing that needs to be done to ensure better prediction. With AI algorithms like neural network getting faster, sleeker and better, one can harness unstructured, big data, both spatial and temporal, find hidden dependencies in the data sets and leverage automated data pre-processing tools with the same cloud infrastructure.
The New Method
Whether its users' online behaviour, browsing patterns, social media usage, typing errors or phone screen resolution or simply ratio of front camera to rear camera images shot can contribute significantly in credit-decisions.
Besides processing cell phone data, AI has enabled the use of many other data sources through techniques like advanced image recognition, Natural Language Processing, voice recognition etc. E.g. Satellite images of rural areas can be used by AI engines to track farms for size, crop type, harvest progress and weather effects which goes into underwriting better farm loans. Customized vehicular loans can be underwritten with flexible terms by deciphering user driving patterns In a country with more than dozens of active languages, NLP can unlock the vernacular dividends by tapping a huge non-English speaking customer base. With nearly half a billion internet users and smartphone devices, AI models have barely scratched the surface of alternative data for user classification.
A big challenge lies in timely revision and enhancement of any data science model. With ever increasing data sources, AI models need to be regularly fine-tuned to account for changing user behaviours, overlapping segment distribution, and varying loan portfolio targets based on different business metrics. e.g. Bias is particularly monitored to avoid already high bureau based credit-score users from forming a larger majority of the portfolio. With advanced self-learning models, this re-calibration becomes easier thereby giving far superior and efficient underwriting results as opposed to traditional credit scoring models. Thus, startups with the requisite Tech expertise in founding teams have a clear competitive edge in scaling up their business and serving newer customer segments with appropriate credit products.
Data
Today, 90per cent of people in India have bank accounts and debit cards, however short-term credit (proxy credit cards) penetration is less than 4per cent, i.e. just about 5per cent population is deemed creditworthy. The lower- and middle-income segment comprises nearly 45per cent of the population (~500M individuals), yet find it difficult to access short-term personal credit from formal financial institutions. Consequently, these individuals have to resort to their personal networks which involve embarrassment and social stigma, or even worse, predatory lenders and loan sharks, who charge exorbitant rates inevitably leading to a debt trap.
One of the clever ways to tap into this credit-starved user base is to pilot small loans of short tenure ("sachet" loans as defined by a PWC report) and inculcate the best practices of credit usage. Researchers at the Centre for Financial Regulation and Inclusion, a South African think tank have expressed that while consumers might use initial loans of small amounts to deal with day-to-day consumption, they develop a "shift in mindset" when they take out larger loans and find ways to purchase items that could ultimately yield profits.