4 Things Data is Not and Where to Focus Instead Data is a raw material, not the finished product.
By Ajay Paghdal Edited by Dan Bova
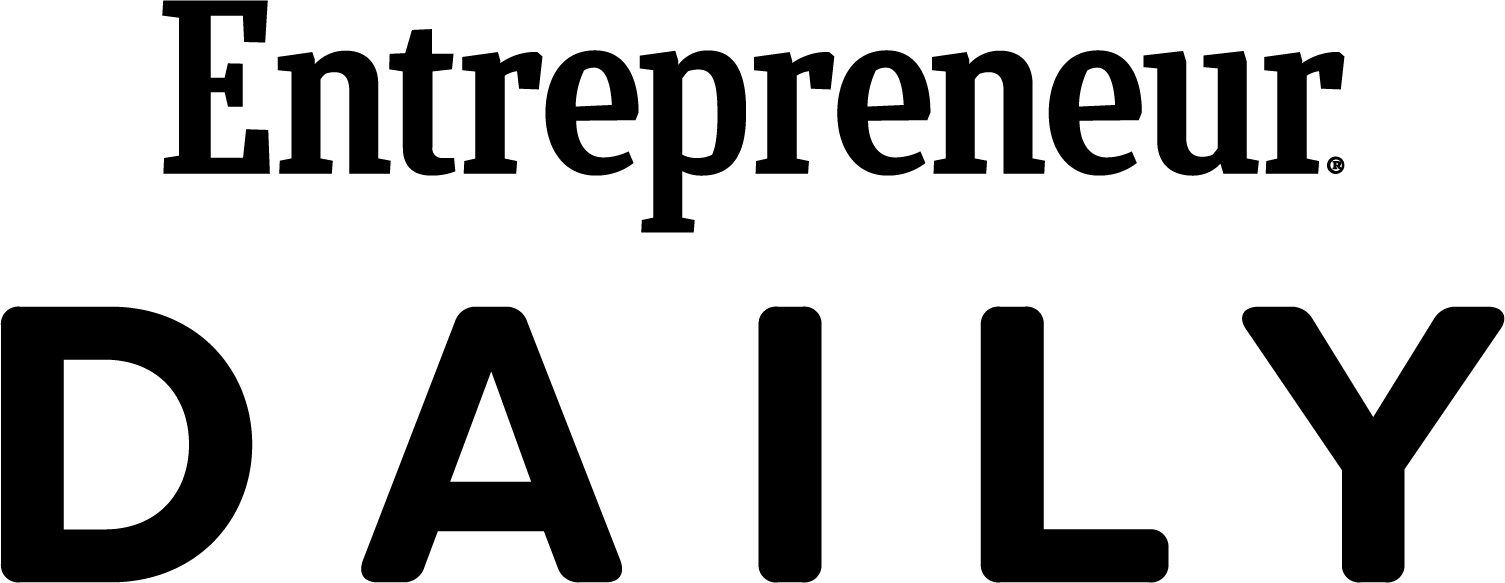
Opinions expressed by Entrepreneur contributors are their own.
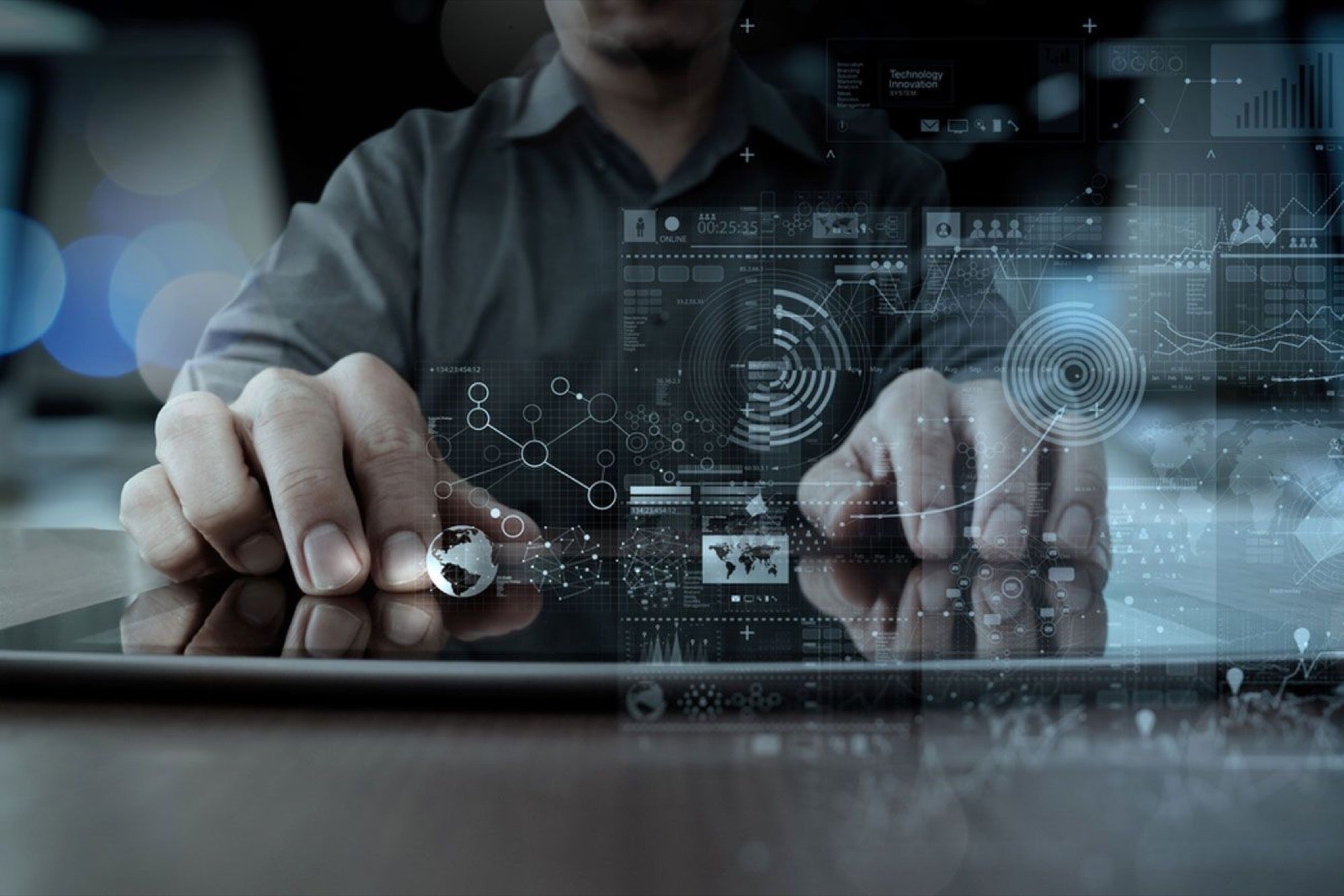
Imagine this scenario. Jane blinks and a smart car pulls up, knowing it's time for her doctor's appointment. No, the smart car isn't connected to Jane's eyes, but through refined data, a company like Google or Uber can know when your next medical checkup is due, help you book an appointment with the doctor and send a smart car to pick you up.
This is the age of data, where the relationship between consumers, advertising agencies and service providers can be strengthened. While we're still far from an utopian world -- where companies have acquired enough intelligence to monetize and use data mined from our lives to allow their machines to interact on an interpersonal level with humans -- it's not too out of reach.
Related: The Big Deal About Big Data For Small Businesses
According to research, each person will generate around 1.7 megabytes of new data every second by 2020. There's value in big data, and everybody wants a piece of the pie. But big data is just part of a huge process. Here's what data is not and how companies should aim to recoup their investment in big data.
1. Data is not cash.
Unlike ad clicks that instantly turns a publisher's or distributor's efforts into cold cash, data is not cash. While companies are hungry for more data, there's already an abundance of data. Data generated is said to reach 44 trillion gigabytes annually by 2020. Extracting the investments venture capitialists have poured into startups in the name of big data is where the job lies.
When your company finds itself at an intersection between providing value and impressing investors, data can make the job of your CEO a lot easier. However, your big data must go through the process where the chief data officer directly gives the CEO recommendations on how best to provide real value while generating revenue from multiple touchpoints made possible by big data.
When there's an abundance of data, getting value from it lies in the job that real humans still have to do.
2. Data is an unprocessed raw material, not a finished product.
Data is not useful unless translated and its value uncovered. The biggest issue with big data is management. Data management under this umbrella involves the following:
- Storage -- As the volume of data a company acquires becomes bigger, it must address the issue of storage. How to keep data safe and protected will be of concern.
- Validating -- Your data still needs to be validated.
- Processing -- The job of the data management team will be to process and make sense of big data.
Big data will need a focused team that is dedicated to processing data generated from IOTs, consumer behaviors and analytics. But the role of this team will be no mean feat as the team needs to make sense of the huge volume of data that will bombard them in real time. Hence, the question will shift from how big is the data being processed to how fast is the data being processed.
Related: 'Big Data' Is No Longer Enough: It's Now All About 'Fast Data'
3. Data is not a quick fix to underperforming marketing.
You will often hear CEOs and CMOs declare big data as part of their strategy. They believe once the company is able to generate enough data through IOT and consumer behavior, investors will begin to smile again. This is a big promise albeit without any real execution plan to follow through.
I hate to break it to you, but data in itself will not solve your performance problems. What will turn an underperforming business around is its approach to addressing key concerns through marketing initiatives supported by data. This means businesses should not be afraid to take the leap into new market segments. It's a no-brainer. Think mobile and augmented reality.
4. Data is not perfect.
From instances where data presents false positives to incorrect findings, data is anything but perfect. Starting from the tools that transmit data to the models on which the analysis is based, it's all prone to error. This is not a result of bad design, per se. Much of the blame lies on the fact that processing big data is a complex job.